Full Seminar Details
Henry Lieberman
Massachusetts Institute of Technology
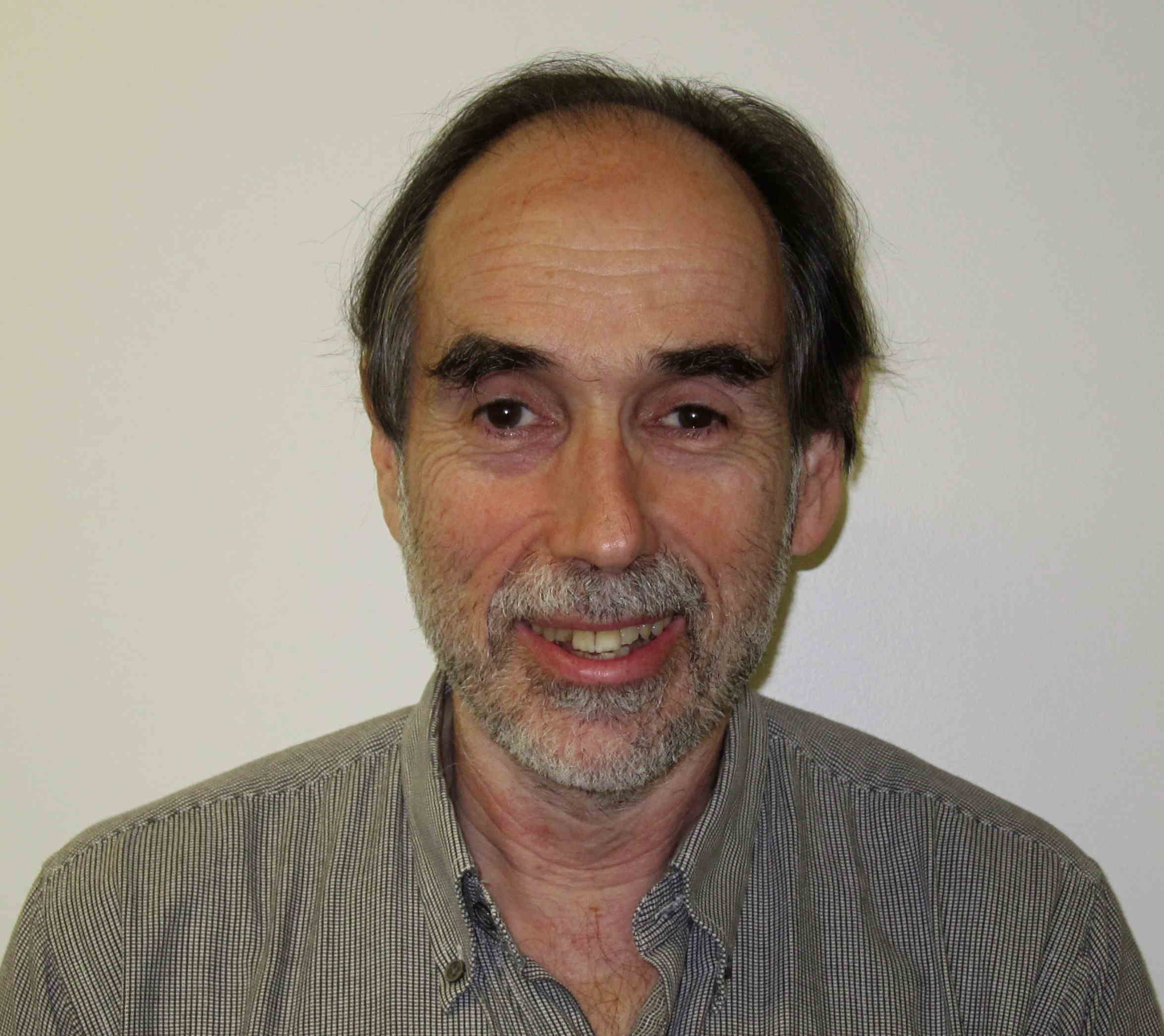
This event took place on Friday 10 November 2017 at 14:00
Story understanding systems need to be able to perform commonsense reasoning, specifically regarding characters’ goals and their associated actions. Some efforts have been made to form large-scale commonsense knowledge bases, but integrating that knowledge into story understanding systems remains a challenge. We have implemented the Aspire system, an application of large-scale commonsense knowledge to story understanding. Aspire extends Genesis, a rule-based story understanding system, with tens of thousands of goal-related assertions from the commonsense semantic network ConceptNet. Aspire uses ConceptNet’s knowledge to infer plausible implicit character goals and story causal connections at a scale unprecedented in the space of story understanding. Genesis’s rule-based inference enables precise story analysis, while ConceptNet’s relatively inexact but widely applicable knowledge provides a significant breadth of coverage difficult to achieve solely using rules. Genesis uses Aspire’s inferences to answer questions about stories, and these answers were found to be plausible in a small study. Though we focus on Genesis and ConceptNet, demonstrating the value of supplementing precise reasoning systems with large-scale, scruffy commonsense knowledge is our primary contribution.
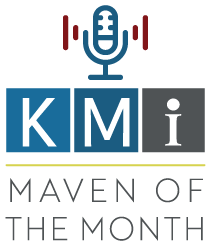
Maven of the Month
We are also inviting top experts in AI and Knowledge Technologies to discuss major socio-technological topics with an audience that comprises both members of the Knowledge Media Institute, as well as the wider staff at The Open University. Differently from our seminar series, these events follow a Q&A format.